Lies, Damn Lies, and Statistics
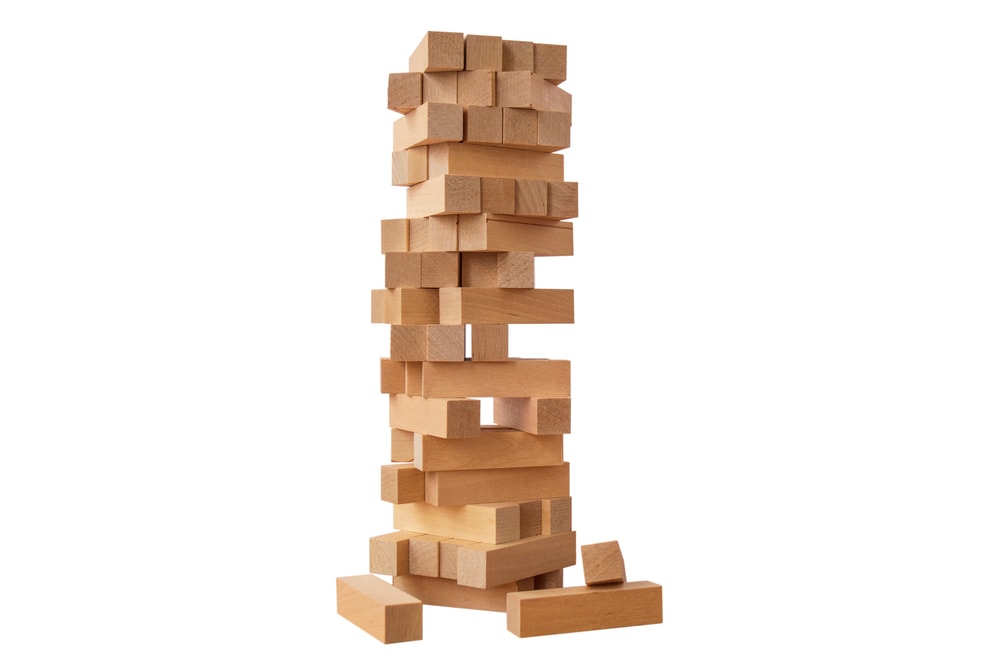
Credit: Shutterstock
Doctors read studies all the time. Based on the study results, they either adopt a new treatment or stop using an old one. Or at least that’s how evidence-based medicine is supposed to work. However, orthopedic hip surgeons just published a really nice article on some new metrics that look at the robustness of clinical results. Frankly, since so much about our medical care system relies on this stuff, every doctor and many patients should understand these basic concepts. Let’s dig in.
Lies, Damn Lies, and Statistics
Mark Twain once said, “There are three kinds of lies: lies, damned lies, and statistics.” For most of us, when we look at the statistics part of a study or hear about some statistical analysis, our eyes glaze over and we begin to think about what’s for dinner. However, in medicine, these concepts are important because major multi-billion dollar decisions that impact patients’ lives are made using statistical analysis. In fact, the old editor of the New England Journal of Medicine, Marcia Angell, M.D., was quoted as saying:
“It is simply no longer possible to believe much of the clinical research that is published, or to rely on the judgment of trusted physicians or authoritative medical guidelines. I take no pleasure in this conclusion, which I reached slowly and reluctantly over my two decades as an editor of the New England Journal of Medicine.”
In part, she said this because of the increased frequency of published studies where statistics were used to make treatments with poor results look like they were helping patients.
P-Values
The basic numbers we doctors pay attention to in a clinical trial testing treatment A against treatment B are p-values. This is basically the probability of randomly obtaining the observed results. For example, if treatment A is 20% better than treatment B, is that just a random finding that wouldn’t happen if you repeated the study, or is it a real difference that would hold up if you did the study a second time? The lower the p-value, the less likely it is that what you’ve observed is due to chance.
However, what we’ll review below are methods used to dig deeper than the p-value. For example, even if the p-value is low and the difference in outcome between treatment A and B is statistically significant, which studies have “barely there” versus robust results?
The Old Way of Determining Clinical Effect
For a doctor or patient reading research studies, there have always been simple ways to tell if the research is showing a big effect that warrants changing how to treat patients or a small effect that doesn’t.
The Size of the Effect
This one is simple. Many readers of studies simply look to see if treatment A is better than treatment B from a statistical standpoint, but never look at the “Effect Size”. For example, treatment A is 10% better than treatment B. In that case, since the new treatment is often much more expensive, you would say that while the difference is statistically significant, it’s not really clinically significant. Compare that to a study that shows that treatment A is 50% better, where it’s very clear that the doctor or patient would want to take a close look at the new therapy.
The Range Overlap
Another problem in studies that show statistically significant differences between the two treated groups can be an overlap in the range of values. While that sounds complex, it’s really not. Any given treatment will generate a range of outcome values. For example, let’s say that we have a 0-10 function scale with 10 being totally normal function and 0 being severely disabled. The range of function numbers in Study 1 for treatment A is 2 to 5 and for treatment B is 3.5 to 7. Hence, there are clearly patients that overlap in both groups who weren’t helped by the treatment. So this isn’t a resounding endorsement.
Now let’s look at Study 2. Here, as shown above, we see a nice separation of the values of the outcome between the two groups. Meaning there is no overlap, so that’s a much bigger endorsement that this study shows a treatment that’s effective.
Responder or “Subgroup” Analysis
I’ve blogged on this one before because it’s used so often in FDA approval trials for new off-the-shelf regen med products. A responder or subgroup analysis” happens when there isn’t a clear difference in the average or mean outcome between the treatment groups. The authors then go back to try to find some subgroup or portion of patients who did respond. For example, something like 40% of the patients had more than a 50% improvement over placebo (while 60% did not). When you see this statistical game being played, it’s almost always a hallmark of poor study results.
The New Way to Look at the Robustness of Clinical Effect
A group of hip surgeons recently published a very nice paper on what they call “Fragility” (1). The general idea is like a game of Jenga. That’s the game where if you remove just a few blocks, the whole tower becomes unstable and falls down. In this case, instead of blocks, you remove a few positive outcomes to see if the stats fall apart.
The authors call these metrics the “Fragility Index and Fragility Quotient”. This is yet another way to look at a study that shows a statistically significant improvement between the groups to see if what you’re looking at is an important treatment or one with “barely there” results. For example, a Fragility Index of 4 means that if you remove just 4 patients who reported improvement from the study, the differences between the groups are no longer statistically different (your p-value collapses). The Fragility quotient works the same way, just using different math.
The author of the new paper used this method to look at all of the research on hip arthroscopy. What did they find? That in most of the studies, removing just a few positive outcomes would have destroyed the stats. In many, if only a few patients who were lost to follow-up (the authors lost track of them) came back with poor outcomes, the study would also fall apart. Hence, the research showing that hip arthroscopy works is fragile and doesn’t show enough robustness to be trusted.
The upshot? I apologize for this detour through a subject that most of us hate. However, for doctors and patients who look at the research to try to find new therapies that may help patients, these are all critical concepts to understand. In addition, these new fragility metrics add a whole new tool that we can all use to find the best evidence-based treatments!
______________________________________________________
(1) Parisien, Robert L. MD1,a; Trofa, David P. MD2; O’Connor, Michaela BA2; Knapp, Brock BA3; Curry, Emily J. BA3; Tornetta, Paul III MD3; Lynch, T. Sean MD2; Li, Xinning MD3 The Fragility of Significance in the Hip Arthroscopy Literature, JBJS Open Access: October-December 2021 – Volume 6 – Issue 4 – e21.00035 doi: 10.2106/JBJS.OA.21.00035
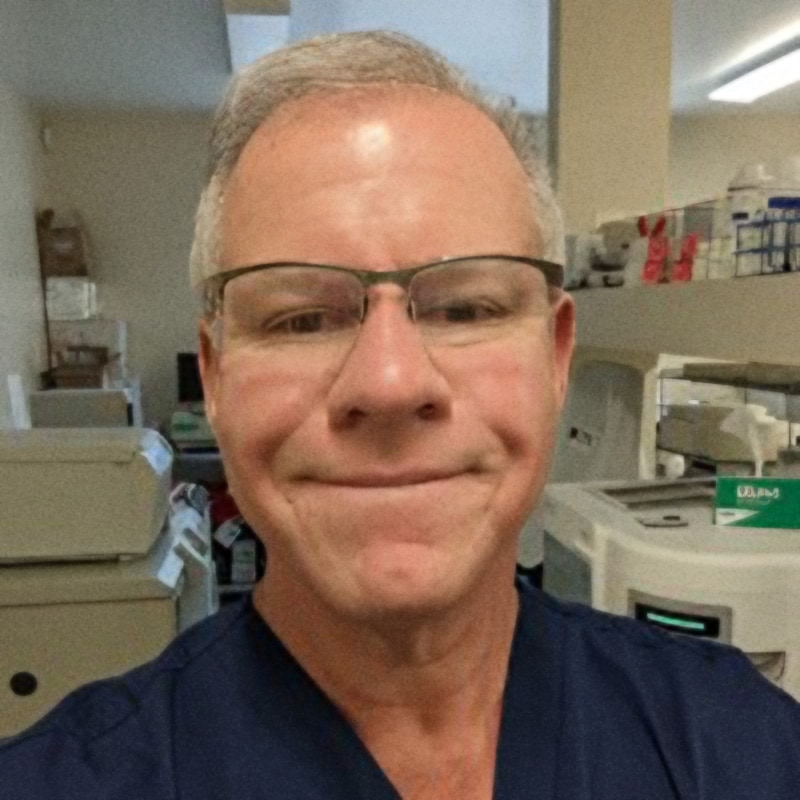
If you have questions or comments about this blog post, please email us at [email protected]
NOTE: This blog post provides general information to help the reader better understand regenerative medicine, musculoskeletal health, and related subjects. All content provided in this blog, website, or any linked materials, including text, graphics, images, patient profiles, outcomes, and information, are not intended and should not be considered or used as a substitute for medical advice, diagnosis, or treatment. Please always consult with a professional and certified healthcare provider to discuss if a treatment is right for you.